The Detection of Atmospheric Rivers in Reanalyses and its Influence on Precipitation
Atmospheric rivers (ARs), or long narrow filaments of enhanced water vapor transport, play a vital role in the hydrologic cycle through their poleward transport of moisture and influence on extreme precipitation and snow and ice melt. Since ARs are a large-scale feature of the atmosphere, their detection is possible in extensive model datasets, and they can then be related to other aspects of the earth system for prediction on numerical weather prediction (NWP), seasonal, and decadal timescales. However, large uncertainties arise due to the numerous methods available for detecting ARs. To reduce ambiguities and inform best practices for future studies in AR science, the Atmospheric River Tracking Method Intercomparison Project (ARTMIP) devised a set of goals and tiered intercomparisons to quantify the characteristics and impacts of ARs and how they vary due to the methodology used for AR detection (https://www.cgd.ucar.edu/projects/artmip/).
ARTMIP began with a Tier 1 intercomparison of AR detection in GMAO’s Modern Era Retrospective analysis for Research and Applications, Version 2 (MERRA-2; Gelaro et al., 2017; Rutz et al., 2019). The Tier 2 intercomparisons build upon the work completed in Tier 1 by aiming to answer more specific science questions. Other Tier 2 intercomparisons are focused on the high-resolution climate catalogs of C20C (Payne et al., 2020) and ARs in a changing climate using CMIP-5 and -6 multimodel ensembles (O’Brien et al., 2021). Here we highlight the reanalysis intercomparison which evaluated the detection of ARs using eleven detection tools (ARDTs) in MERRA-2 alongside the European Centre for Medium-Range Weather Forecasts’ ERA5 (Hersbach et al., 2020) and the Japan Meteorological Agency’s JRA-55 (Kobayashi et al., 2015).
ARDTs use variety of methods to detect ARs, including absolute and relative thresholds, geometry thresholds, deep learning, and object identification, but nearly all use integrated water vapor transport (IVT) as an input field. MERRA-2 has an elevated climatology of total precipitable water vapor, and therefore IVT, compared to ERA5 and JRA-55. Therefore, ARDTs that utilize an absolute threshold, such as the threshold of 500 kg m-1 s-1 used by Reid et al. (2020), tend to detect more frequent ARs in MERRA-2 than the other reanalysis products. An example of this is demonstrated in the figure below which shows the fraction of ARDTs that detected an AR during an event from November 2014. All participating ARDTs detected the AR over the Pacific Ocean in MERRA-2, which was not the case for ERA5 or JRA-55. The finer resolution of ERA5 results in narrower ARs stemming from more intense gradients in the field of IVT.
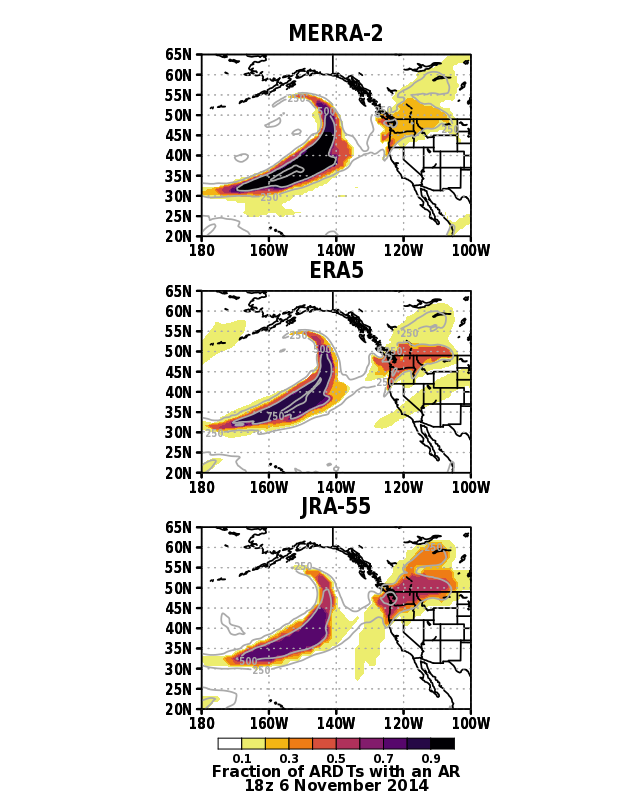
A well-documented region for ARs is the northeast Pacific, with ARs making landfall on the west coast of the United States and British Columbia. Figure 2 shows the annual mean precipitation associated with ARs detected in the reanalyses by at least half of the ARDTs within the time period of 1980 through 2019. An independent observational dataset for precipitation was used, the Climate Prediction Center Unified (CPCU) gauge-based analysis of daily precipitation (Chen and Xie, 2008). Prior to computing any statistics, the precipitation observations were regridded to match the resolution of the individual reanalyses.
The overall spatial patterns and magnitudes for the annual mean precipitation associated with ARs in MERRA-2, ERA-5, and JRA-55 are similar due to the influence of topography on precipitation (Figure 2a-c). Differences in the spatial resolution are evident as the field is smoothed in JRA-55 especially relative to ERA-5. Along the Sierra Nevada Mountains, there is more precipitation associated with ARs in MERRA-2 than ERA5 and JRA-55. ARDTs with strict thresholds tend to result in high values for the mean precipitation associated with an AR as more intense events are included in the average. Given that more ARs are generally detected in MERRA-2, this is somewhat counter-intuitive. This region is, however, located at a gradient where ARDTs begin to no longer detect the inland penetration of ARs. Though difficult to say for sure, it can be hypothesized that the intensity distribution of consensus ARs detected in MERRA-2 in the Sierra Nevada Mountains includes fewer weaker ARs and more intense ARs than ERA5 and JRA55.
Another possibility for the higher mean precipitation in MERRA-2 is that a few ARDTs only submitted AR tags in ERA5 for the 20-year period beginning in 2000, which was the minimum in order to participate in the project. Embedded within the results for precipitation is the temporal trend in precipitation for the region, particularly in southern California and the desert southwest. This is manifested through a lower mean precipitation from ARs (Figure 2b), higher percentage of precipitation resulting from ARs (Figure 2e), and more precipitation associated with consensus ARs than the ensemble means (Figure 2b).
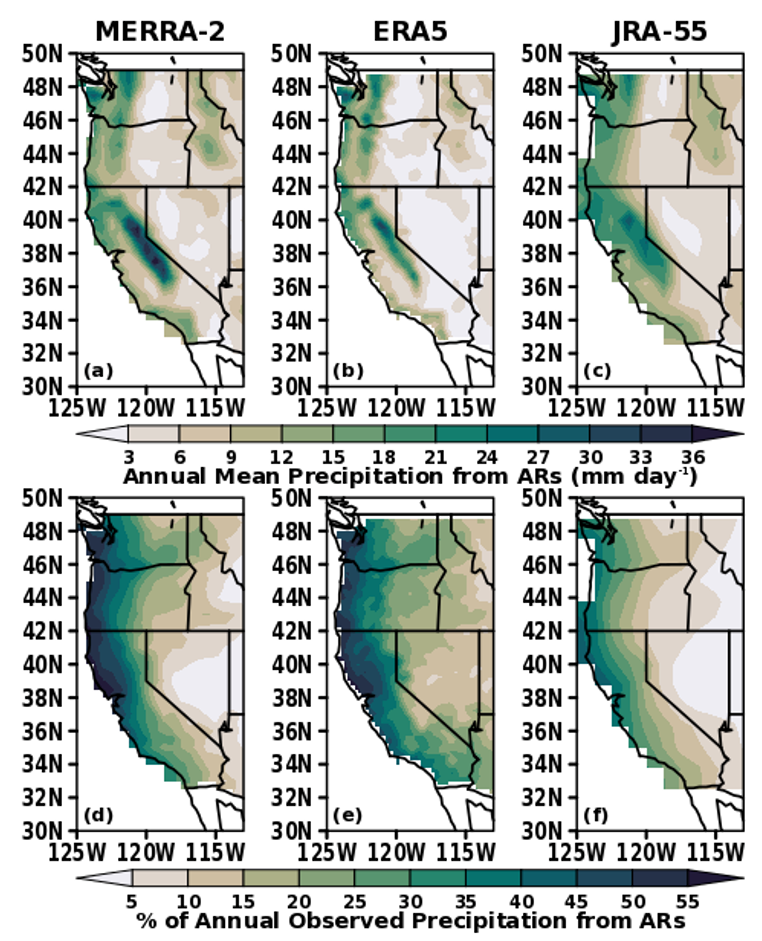
Additional results from the ARTMIP Tier 2 Reanalysis Intercomparison are available in Collow et al. (2022), which was recently highlighted by EOS (https://eos.org/editor-highlights/comparing-methods-for-analysis-of-atmospheric-rivers).
References
Chen, M., & Xie, P. (2008). CPC unified gauge-based analysis of global daily precipitation. Western Pacific Geophysics Meeting. American Geophysical Union. Abstract A24A-05.
Collow, A. B. M., Shields, C. A., Guan, B., Kim, S., Lora, J. M., McClenny, E. E., et al. (2022). An overview of ARTMIP's Tier 2 Reanalysis Intercomparison: Uncertainty in the detection of atmospheric rivers and their associated precipitation. Journal of Geophysical Research: Atmospheres, 127, e2021JD036155. https://doi.org/10.1029/2021JD036155
Gelaro, R., McCarty, W., Suárez, M. J., Todling, R., Molod, A., Takacs, L., et al. (2017). The modern-era retrospective analysis for research and applications, Version 2 (MERRA-2). Journal of Climate, 30(14), 5419–5454. https://doi.org/10.1175/JCLI-D-16-0758.1
Hersbach, H., Bell, B., Berrisford, P., Hirahara, S., Horányi, A., Muñoz-Sabater, J., et al. (2020). The ERA5 global reanalysis. Quarterly Journal of the Royal Meteorological Society, 146, 1999–2049. https://doi.org/10.1002/qj.3803
Kobayashi, S., Ota, Y., Harada, Y., Ebita, A., Moriya, M., Onoda, H., et al. (2015). The JRA-55 reanalysis: General specifications and basic characteristics. Journal of the Meteorological Society of Japan, 93, 5–48. https://doi.org/10.2151/jmsj.2015-001
O’Brien, T. A., Wehner, M. F., Payne, A. E., Shields, C. A., Rutz, J. J., Leung, L. R., et al. (2021). Increases in future AR count and size: Overview of the ARTMIP Tier 2 CMIP5/6 Experiment. Earth and Space Science Open Archive, 31. https://doi.org/10.1002/essoar.10504170.1
Payne, A. E., Demory, M.-E., Leung, L. R., Ramos, A. M., Shields, C. A., Rutz, J. J., et al. (2020). Responses and impacts of atmospheric rivers to climate change. Nature Reviews Earth & Environment, 1(3), 143–157. https://doi.org/10.1038/s43017-020-0030-5
Reid, K. J., King, A. D., Lane, T. P., & Short, E. (2020). The sensitivity of atmospheric river identification to integrated water vapor transport threshold, resolution, and regridding method. Journal of Geophysical Research: Atmospheres, 125, e2020JD032897. https://doi.org/10.1029/2020JD032897
Rutz, J. J., Shields, C. A., Lora, J. M., Payne, A. E., Guan, B., Ullrich, P., et al. (2019). The Atmospheric River tracking method intercomparison project (ARTMIP): Quantifying uncertainties in Atmospheric River climatology. Journal of Geophysical Research: Atmospheres, 124, 13777–13802. https://doi.org/10.1029/2019JD030